This post first appeared on IBM Business of Government. Read the original article.
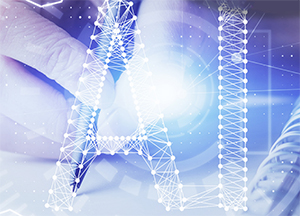
Leveraging technology with speed and reliability has never been as important as in today’s world of distance work, virtual meetings, and IT development via geographically dispersed teams.
As economies and societies transform in response to COVID-19 and its aftermath, governments in the US and around the world seek innovations that enable them to interact with the public, industry, and each other — addressing both immediate social and economic needs for services and longer-term imperatives for operational effectiveness.
Managing risk when introducing new technologies will be fundamental to sustaining their success in light of constant change. A risk management framework will allow agencies to assess benefits from introducing emerging “intelligent automation” technologies powered by artificial intelligence (AI), while controlling downsides from security, cost, workforce, and other perspectives.
Our Center continues to engage in research about key considerations for managing risks from AI implementation that can guide decisionmaking across the broader suite of intelligent automation (including robotics process automation, blockchain, open source, and hybrid cloud computing), and we have new research in the pipeline. In this next installment of our series on enabling the government to address risks from the current transformation responsibly, we highlight the most important of those considerations.
Important questions for review in managing risk from AI that apply more broadly to emerging technologies, including:
- How should government address risks that vary with different technology use and maturity?
- What principles or guidelines should agencies follow to address design and implementation issues such as ethics, bias, transparency, data quality, and cybersecurity?
- How might agencies transition from a culture of risk avoidance to one of risk tolerance?
Different Uses of Technology Bring Different Risks
In the Center’s recent report Risk Management in the AI Era: Navigating the Opportunities and Challenges of AI Tools in the Public Sector, authors Justin Bullock and Matthew Young note that agency decisions about AI implementation depend on the programmatic goal being advanced by the technology. Government may yield more effective results through a three-part framework where AI risks vary based on the need for human discretion, as shown in the chart below:
As the complexity of the work increases, so does the need for introducing technologies that are more effective in risk mitigation – the report authors note that “seizing the opportunities and mitigating the hazards associated with adopting AI tools requires paying careful attention to the match between technology and task.” This continuum-based approach can help guide government toward which tasks can be quickly automated, and which require more planning and interaction with industry and academic partners.
The Government of Canada uses a risk assessment framework to help guide agencies in making decisions along a similar continuum. As discussed in More Than Meets AI: Building Trust, Managing Risk, the Center’s 2019 report with the Partnership for Public Service, Canada developed an Algorithmic Impact Assessment. The tool rates proposed projects from 1 to 4, with 1 as low impact level and 4 as high, and identifies the different technology and control strategies used at each level. These ratings are all available online to foster transparency, and each rating level is associated with a different set of agency.
Addressing Design and Implementation Issues
Reducing risk from AI and related applications relies in large part on designing algorithms that are “explainable.” Many machine learning models are difficult to interpret, making it hard to understand how a decision was made (often referred to as “black box” decision). Another issue arises when low quality data (i.e., data that embeds bias or stereotypes or simply does not represent the population) is used in un-interpretable models, making it harder to detect bias. On the other hand, well-designed, explainable models can increase accuracy in government service delivery, such as a neural network that could correct an initial decision to deny someone benefits for which they are entitled.
Research into interpretability of models can help reduce risk and build trust in AI. More broadly, educating stakeholders about AI – including policy makers, educators, and even the general public — would increase digital literacy and provide significant benefits. Government, industry, and academia can work together in explaining how sound data and models can both inform the ethical use of AI.
The recent Center report from authors Bullock and Young offers a risk reduction framework for adoption of AI tools that has relevance for a broader suite of technologies. This framework addresses multiple criteria, including effectiveness, efficiency, equity, manageability, and political legitimacy.
These and similar considerations can be built into systems in an auditable way. In a recent article for The Business of Government magazine entitled “Algorithmic Auditing: The Key to Making Machine Learning in the Public Interest,” Harvard Kennedy School writer Sara Kassir presented an approach to ensure that AI and related applications include risk reduction as part of an auditable systems development process. As Kassir wrote, “because algorithmic audits encourage systematic engagement with the issue of bias throughout the model-building process, they can also facilitate an organization’s broader shift toward socially responsible data collection and use.”
Moving From Risk Avoidance to Risk Tolerance
Governments will see an AI-powered transformation of technologies occur over the next 15 to 30 years. However, as noted during the 2019 Roundtable, agencies face several challenges in educating their workforce on how to adapt to new opportunities and risk in this evolution:
- Lack of training and skills
- Lack of a comprehensive AI strategy
- Lack of engagement with academic experts
- Gaps in existing policies in government, particularly around risk classification
- Access to data, specifically a lack of inter-agency data sharing
- Barriers to procurement
Building a culture of risk tolerance in introducing new technologies can be facilitated by focusing on a cost-benefit framework for considering social issues like ethics. Such an approach would allow agencies to compare the risks associated with AI (e.g., potential for human harm, discrimination, funds lost) with the benefits (e.g., lives saved, egalitarian treatment, funds saved) throughout the lifecycle of an algorithm’s development and operation. Cost-benefit analyses often include scenario planning and confidence intervals, which could work well in building a business case for AI systems over time—provided that the “costs” considered include not only quantifiable financial costs, but more intangible, value-based risks as well (such as avoiding bias or privacy harms). This could demonstrate a clear way to communicate risk and decisions about how and when to use AI — including risks of leveraging AI to support a decision, relative to risks of decisions based solely on human analysis – in a way that informs public understanding and dialogue.
In addition, ensuring confidence in data used by AI and other automated decision systems makes proper data governance necessary – including a focus on data quality, security and data user rights, and ensuring that automated decision systems are not discriminatory. Another element of data governance could set-up protocols for inter-agency data sharing, which can increase efficiency and support. This could also introduce privacy risk, but using a risk management perspective allows for agencies to assess how much personal data they need to collect and store based on the benefits to the data subjects.
Conclusion
Government employees often lack familiarity with AI and related technology, and are understandably skeptical about its impact on their work. Greater engagement across agencies in setting forth needs and priorities, defining factors that can promote trust in systems, and developing pathways to explain the technology, could enhance acceptance across the public sector.
Sharing best practices is especially important given the differential levels of maturity in AI use across agencies and even levels of government. Addressing risk in AI and other emerging technologies will require a multi-disciplinary approach that brings people with different expertise (such as engineers and lawyers) to the same table. With greater understanding of and involvement in the technology, agencies can promote the understanding of benefits and risks and foster a cultural shift in supporting responsible adoption.
A perfect AI system, one free of risk, will never be actualized. The more practical path forward in understanding and addressing underlying risk is to engage early with developing AI prototypes, iterate on the solutions, and learn from the results. In fact, the risk of not using AI may be greater than any risk from responsible and ethically designed systems. Risk-based approaches that include public dialogue provide a starting point.
As agencies adjust to the current and future imperatives that are accelerated by COVID recovery and response, they will increasingly rely on intelligent automation – led by AI and including other emerging technologies — for developing policy, implementing programs, and providing services and information. In this context, government leaders and stakeholders must understand and act on methods for making risk-based decisions based on benefits and costs of technology adoption. These and similar strategies will help agencies recognize and communicate the impacts of innovation, at a time where such innovation is critical to successful performance by government in serving the nation.